4 Problems with AI That Remain Unsolved
Explore 4 critical challenges confronting AI today: from ethical dilemmas to unsupervised learning challenges.
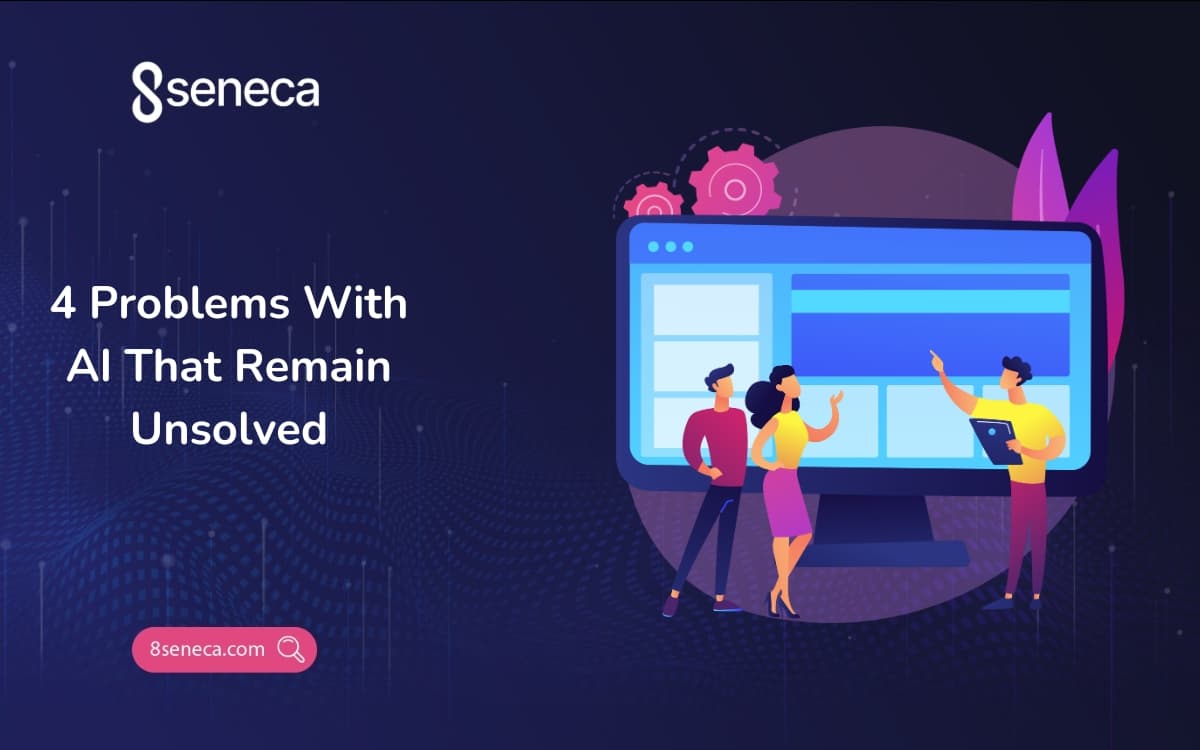
Artificial Intelligence has made remarkable strides in recent years. It has revolutionized industries and reshaped the way we live and work. However, amidst the excitement surrounding its advancements, there are several significant problems that remain unsolved. These issues pose challenges to its widespread adoption and ethical implementation. In this article, we’ll explore 4 problems with AI that remain unsolved.
Ethical Concerns
Bias in AI Algorithms
One of the most prevalent issues haunting artifical intelligence is the insidious presence of bias within its algorithms. artifical intelligence systems are trained on vast datasets, often reflects ssocietal prejudices and systemic inequalities. As a result, these biases spread through the decision-making of AI systems, making discrimination worse and widening gaps in society.
Also, bias in AI algorithms can show up in different ways. It can be clear discrimination against specific groups or more hidden biases based on cultural stereotypes or past prejudices. These biases affect decisions made by AI in things like hiring, lending and criminal justice. This keeps systemic inequalities going and pushes vulnerable groups to the sidelines.
Additionally, AI can make existing unfairness worse by turning biased practices into rules. For instance, biased algorithms used in predictive policing might focus too much on minority neighborhoods, leading to unfair surveillance and too much policing. Similarly, biased hiring algorithms can keep gender or racial differences in job chances going, making existing discrimination stronger.
Lack of Transparency
The fact that AI algorithms are hard to understand makes it tough for people to trust them. A lot of AI models work in ways that are like black boxes, so it’s hard for users to know how decisions are made. This lack of clarity doesn’t just make it harder to hold anyone responsible but also makes people worry about whether AI decisions are fair and ethical.
Moreover, the lack of transparency in AI algorithms complicates efforts to hold developers and stakeholders accountable for their actions. Without visibility into the decision-making processes of AI systems, it becomes challenging to identify and rectify instances of bias, errors or unethical behavior. Consequently, developers may evade responsibility for the consequences of AI-driven decisions, thus leads to a lack of accountability and oversight.
Being clear about how AI works is really important for making people trust it and making sure it’s fair. Users have to know how artifical intelligence makes decisions so they can tell if they’re fair and real. If AI isn’t clear, people might stop trusting it. They might doubt it, fight against it and eventually, stop believing in technology as a solution.
Accountability Issues
Deciding who’s responsible for what AI does is a big argument. As AI gets more independent, it’s harder to figure out who should take the blame. Should it be the people who made it, the data it used or the algorithm itself? Solving these responsibility problems is important for making people trust AI and making sure it’s used ethically.
Also, because there aren’t clear laws or rules about artifical intelligence, it’s even harder to figure out who’s responsible. Even though developers should make and use AI responsibly, there aren’t many rules to make sure they do. Setting up clear laws and rules is important. It helps say who’s responsible for what, sets up good ways of doing things and stops people from using AI in bad ways.
Even though AI can do a lot on its own, people still need to keep an eye on it. People have to watch what AI does, find any mistakes or unfair things and step in if needed to stop bad outcomes. When people stay involved in how AI is used, it makes sure it’s used fairly and ethically.
Limitations in Generalization
AI systems often struggle to transfer knowledge from one domain to another. This limitation hampers their ability to generalize learning across diverse contexts. While AI may excel in specific tasks or domains, its capacity to apply knowledge to unfamiliar situations remains rudimentary. This constraint hinders its adaptability and scalability.
Furthermore, artifical intelligence systems tend to acquire domain-specific expertise rather than generalizable knowledge. For example, a machine learning model trained on medical imaging data may excel at diagnosing specific diseases but may struggle to apply its knowledge to other medical specialties or unrelated domains. This lack of generalization poses challenges for deploying artifical intelligence across diverse applications and industries.
Moreover, AI systems often struggle to grasp nuanced contextual cues, such as sarcasm, tone, or cultural nuances which are essential for accurate decision-making. Consequently, AI may misinterpret or overlook critical contextual information, leading to errors in judgment and outcomes. Addressing these contextual understanding difficulties is crucial for improving the generalization capabilities of AI systems and enhancing their real-world applicability.
Unsupervised Learning Challenges
Data Inefficiencies
Unsupervised learning is a big part of artifical intelligence. It tries to learn from data that doesn’t have labels, without someone telling it what to look for. But sometimes, this way of learning has problems with the data it gets and needs a lot of it to find useful stuff. Also, how good the data is really matters for unsupervised learning to work well. This makes it hard to use in real life.
Also, AI systems might come across unfairness or unevenness in the data they use to learn, which can make the way they understand the data not right or wrong. Biased data can make it hard for AI systems to understand things well, because they might learn wrong connections or miss important things in the data. Fixing data bias and unevenness is really important for making sure AI works well in lots of different situations.
Learning Without Explicit Supervision
While unsupervised learning holds promise for discovering latent patterns and structures within data, it lacks the guidance provided by explicit supervision. Consequently, AI systems may struggle to discern meaningful insights from noisy or ambiguous data. This struggle hampers their ability to make accurate predictions and decisions. Developing techniques for guiding and shaping unsupervised learning processes is crucial. It helps in improving the generalization capabilities of AI systems and enhancing their utility in real-world scenarios.
Also, unsupervised learning algorithms can be affected by unusual data points. These unusual points can change the way the artifical intelligence learns and make it not work well. Making sure AI can handle unusual data needs good techniques to prepare the data. It also needs improvements to the algorithms to find and fix the effect of unusual data points. Fixing these problems is really important for making unsupervised learning work better in real-life situations.
Interpretability and Explainability Challenges
Black Box Nature of AI Models
Many AI models operate as black boxes, meaning that their internal workings are opaque and difficult to interpret. This lack of interpretability poses significant challenges in understanding how AI arrives at its decisions, which is critical for ensuring transparency and accountability.
The black box nature of AI models undermines trust and confidence in AI-driven systems. Users are reluctant to rely on artifical intelligence recommendations or decisions when they cannot understand the rationale behind them. Consequently, the lack of interpretability hinders the widespread adoption of AI solutions in critical domains such as healthcare, finance, and criminal justice.
Furthermore, the opacity of artifical intelligence models complicates regulatory compliance efforts, particularly in highly regulated industries such as healthcare and finance. Regulators require transparency and accountability in algorithmic decision-making processes to ensure fairness, non-discrimination and compliance with legal and ethical standards. However, achieving regulatory compliance is challenging when AI operates as a black box.
Explainability Techniques
Addressing the lack of interpretability in AI models requires the development and adoption of explainability techniques that shed light on their decision-making processes. Explainability techniques aim to provide insights into how AI arrives at its decisions, making them more transparent and interpretable for users.
One common explainability technique is feature importance analysis. This method identifies the most influential features or factors driving AI predictions or decisions. By understanding which features are most relevant to AI outcomes, users can gain insights into the underlying decision-making process and assess the validity and reliability of AI predictions.
Another approach to explainability is model visualization. This technique visualizes the internal structure and behavior of AI models in an interpretable manner. Model visualization techniques provide intuitive representations of complex AI models. They enable users to understand their decision-making logic and identify potential sources of bias or error.
Trade-Offs Between Accuracy and Interpretability
A key challenge in achieving interpretability is balancing the trade-off between model accuracy and interpretability. In many cases, increasing model interpretability may come at the expense of predictive performance. Therefore, developers must carefully navigate this trade-off to ensure that artifical intelligence models are both accurate and interpretable, striking a balance between transparency and performance.
Moreover, there is a fundamental trade-off between model complexity and interpretability. Complex artifical intelligence models such as deep neural networks may achieve high accuracy but are often difficult to interpret due to their intricate architectures and non-linear decision boundaries. Simpler models, on the other hand, may sacrifice some predictive performance but are more interpretable and easier to understand.
Conclusion
In conclusion, while artifical intelligence holds immense promise for transforming industries, there are significant challenges persist on the path to its realization. From ethical concerns surrounding bias and transparency to limitations in generalization and the quest for interpretability, addressing these challenges is essential for the responsible and ethical deployment of artifical intelligence. By tackling these unsolved problems, we can harness the full potential of artifical intelligence while safeguarding against unintended consequences.
At 8Seneca, we’re really good at providing personalized B2B services, especially in IT outsourcing solutions. If you need help with IT outsourcing, don’t hesitate to reach out to us. Also, we’re looking for enthusiastic interns to join our team. Check out our recruitment center for more information about the roles we have available.
Related Articles
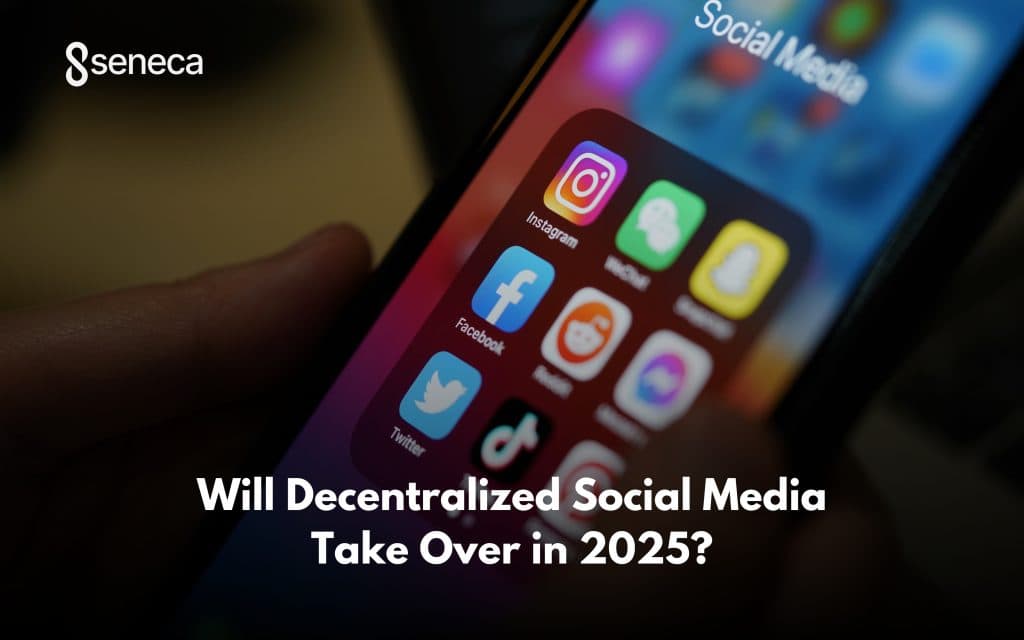
Jan 14, 2025
Read more
Will Decentralized Social Media Take Over in 2025?
Explore if decentralized social media could reshape online interactions in 2025 with greater privacy and control.
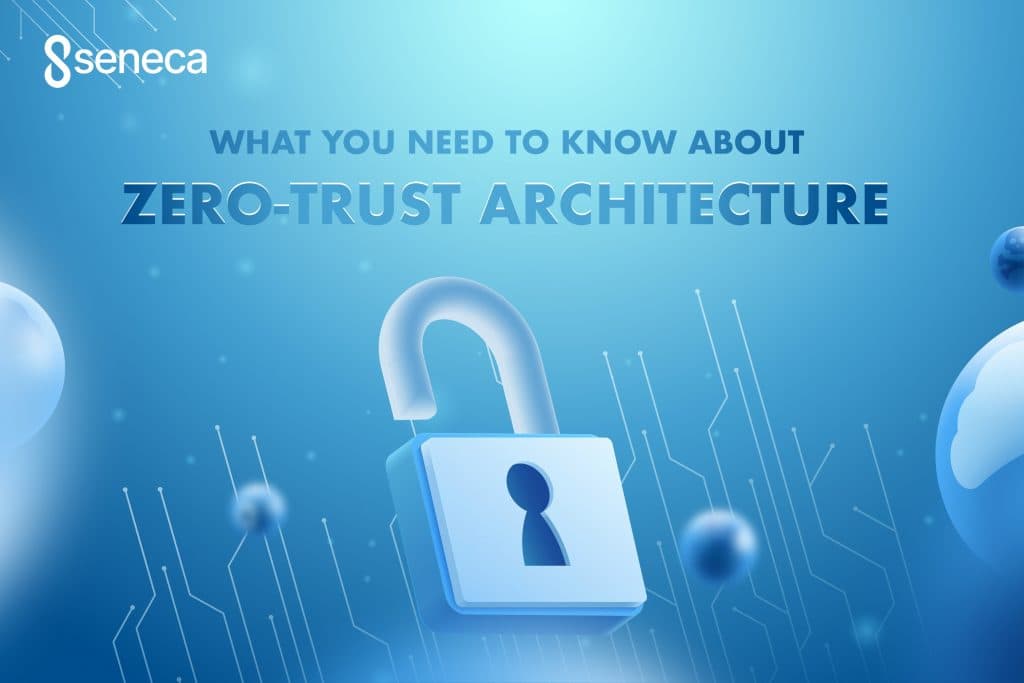
Jan 08, 2025
Read more
What You Need to Know About Zero-Trust Architecture
Learn the essentials of Zero-Trust Architecture: how it enhances cybersecurity by verifying every user and device.
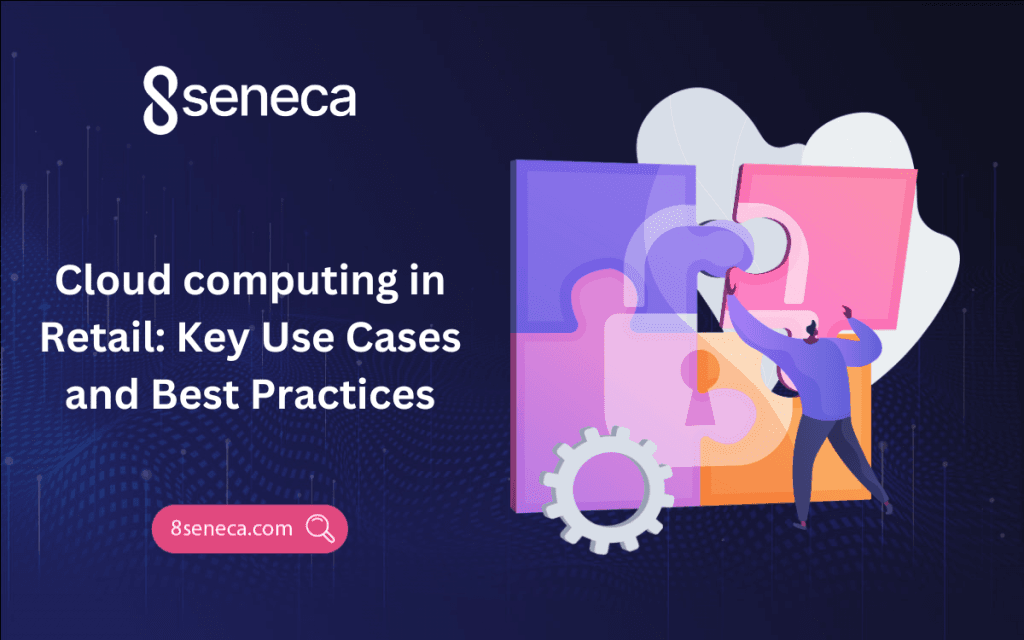
Oct 07, 2024
Read more
Cloud Computing in Retail: Key Use Cases and Best Practices
Cloud computing helps retailers optimize operations, streamline inventory, and improve decision-making with real-time data.
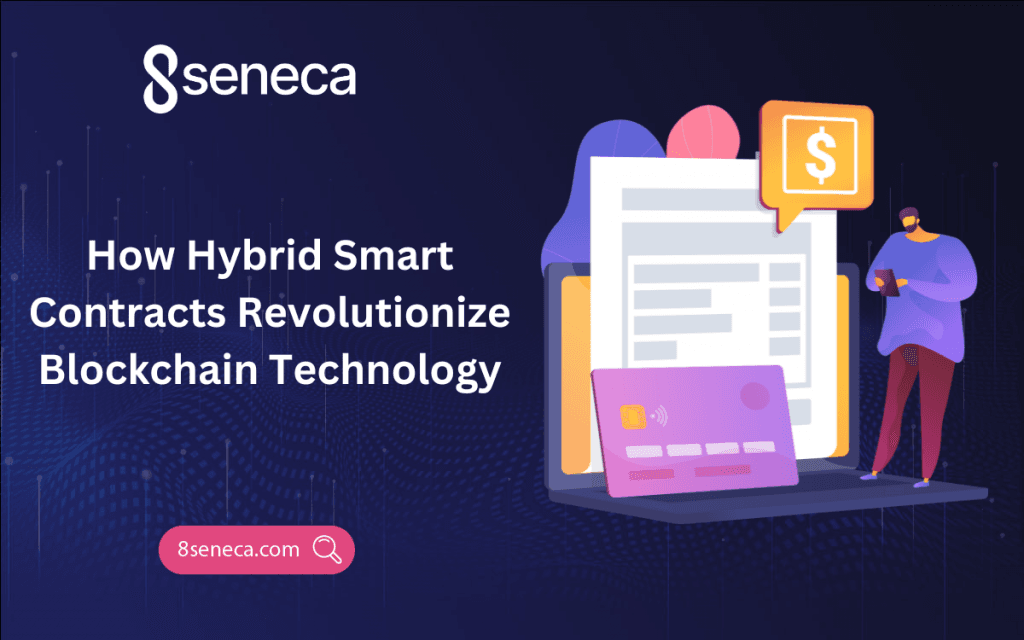
Sep 27, 2024
Read more
How Hybrid Smart Contracts Revolutionize Blockchain Technology
Discover what hybrid smart contracts are and how they are revolutionizing blockchain technology, including key benefits and challenges.
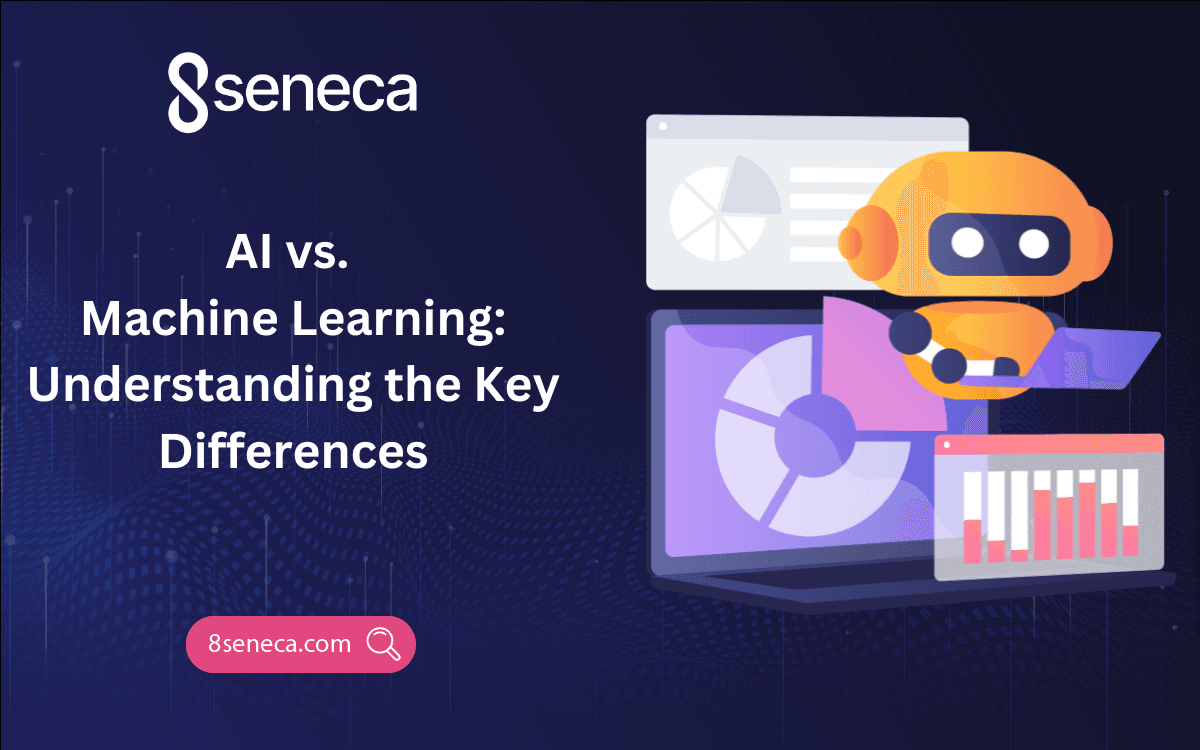
Sep 25, 2024
Read more
AI vs. Machine Learning: Understanding the Key Differences
Learn the differences between AI and ML, their impact on business, and how to leverage them effectively in business.
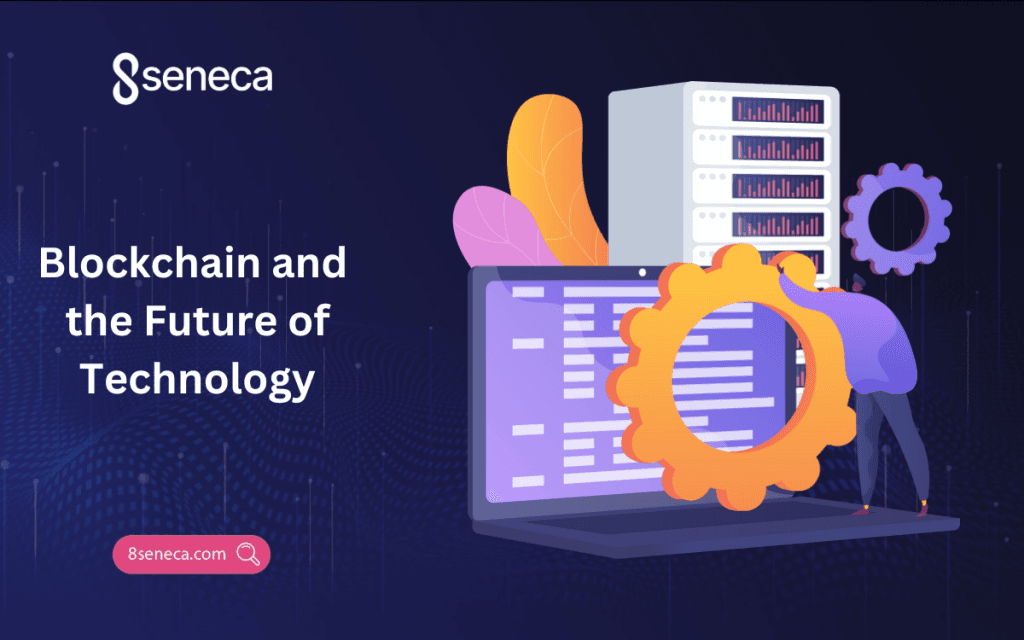
Aug 14, 2024
Read more
Blockchain and the Future of Technology
Explore how blockchain technology is revolutionizing industries like finance, healthcare, and supply chain management.